Predictability and Prediction Research
- Home
- Academics
- Departments & Units
- Atmospheric, Oceanic & Earth Sciences
- Research
- Predictability and Prediction Research
Admission CTAs
Main navigation
Section Navigation: Research
Main navigation
Predictability and Prediction Research
Can we predict the weather two weeks from today? Two months from today? Two years from today? Two decades from today?
These are the kinds of questions we study at George Mason's Department of Atmospheric, Oceanic, and Earth Sciences. Of course, it is not possible to predict the specific weather beyond two weeks, but we can predict the probability of certain weather events over extended periods of time. Our research studies the predictability of global phenomena on a range of time scales. We use global climate and weather models, machine learning, and advanced statistical techniques to perform this research.
Faculty:DelSole, Dirmeyer, Huang, Kinter, Pegion, Shukla, Stan, Straus, Cash
Subseasonal to Seasonal Predictions
Skillful Subseasonal Predictions Are Possible
AOES faculty member DelSole and collaborators showed that the average temperature over certain parts of the United States could be predicted out to weeks 3-4 (DelSole et al., 2017). Ordinarily, the probability of above or below normal temperature is 50-50, just like predicting heads on a coin flip. Using a forecast model, above or below normal temperatures could be predicted correctly 67 percent of the time-- much better than a coin flip.
News Article: Breaking New Ground in Weather Forecasting
Discovering New Sources of Subseasonal Predictability
The most significant source of subseasonal weather predictability in the United States stems from sea surface temperatures, particularly the El Nino Southern Oscillation. Are there undiscovered factors at play that could be shaping our weather in ways we have not grasped yet? DelSole and his students have been pioneering a novel approach that doesn't rely on preconceived mechanisms. Specifically, they have been using a technique known as Canonical Correlation Analysis (CCA). CCA is like a detective searching for correlations between temperature fields across the US, but even better than a detective because CCA is guaranteed to find the patterns with the largest correlation. Unlike machine learning, CCA can find robust correlations in relatively small data sets. This means that if CCA cannot find a correlation, then nothing can. Once a predictable temperature signal is identified, the real investigation starts. Where does this predictability come from? Is there a hidden factor at work? The answers to these questions could improve our ability to predict weather weeks in advance.
Understanding the Limits of Predictability
Weather forecasts become less reliable as we project further into the future, leaving us pondering: what is the ultimate limit of predictability? Among the myriad of questions, one stands out-- what can we predict the longest, and just how far can we see into the future of our climate? Dr. DelSole and his team are exploring this question, utilizing linear stochastic models to dissect the climate system's predictability. These models, a fusion of linear dynamics and stochastic forcing, generate forecasts across various time scales-- from subseasonal to decadal-- that rival the accuracy of the most advanced climate models today. The behavior of stochastic models can be expressed in terms of modes (also called eigenmodes), similar to the way that a vibrating string can be expressed in terms of fundamental tones. While each mode has a limited window of predictability, the magic happens when we combine them. This combination can extend predictability far beyond the limits of any single mode. Imagine knowing that the most predictable mode fades after a year. How much further could we push our foresight with the right combination of these modes? Two years, five years, or more? What's the science behind this extension in predictability? These are the mysteries DelSole's team is dedicated to unraveling.
The relative roles of decadal climate variations and changes in the ocean observing system on the ENSO seasonal prediction skill
Using the 60-year seasonal reforecast dataset, Huang et al. (2021) examined the temporal variations of the ENSO prediction skill during 1958–2016 in the context of the evolution in the tropical Pacific subsurface ocean observing system. To examine the temporal variations of the seasonal prediction skill, spatial correlation skill (SCS) of the predicted SST anomalies (SSTA) in the tropical Pacific Ocean within 10S-10N and temporal correlation skill (TCS) of the area-averaged SSTA throughout the same basin for the four periods of 1958–1978, 1979–1994, 1995–2005 and 2006–2016 were evaluated. These periods correspond to low amount, first increase, medium amount and second increase of the subsurface ocean temperature observations. It is shown that the influence of the observing system is detectable in the skill increase during the period 1995–2005. However, the impact of the subsurface ocean observing system is difficult to quantify in the prediction skill metrics during 2006–2016. Overall, SCS is determined largely by the magnitude of the observed SSTA in the target month. There is visible skill increase in the TCS before and after 1979, but this appears to be the result of variations in the properties of the observed SST. Thus, potential impacts of the observing system are masked by climate variations of SST at decadal time scales. In particular, the multidecadal modulations of the tropical Pacific SST associated with the climate shifts in the late 1970s and the early 2000s have more significant influence on prediction skill than the changes in observing system.
The Unified Forecast System
The NOAA National Weather Service is developing a next-generation forecast model, called the Unified Forecast System (UFS). Several AOES faculty, scientists, post-docs and students are involved in developing and validating the performance of UFS.
AOES faculty member Dirmeyer is leading several efforts to ensure forecast models such as UFS represent the feedbacks from land to atmosphere correctly (Seo et al. 2023), including a new project in collaboration with NCEP and NCAR to couple a global version of the National Water Model to UFS to link the land and ocean for the first time in a weather forecast model.
AOES faculty member Kinter is helping to lead the transition from research to operations of the next generation UFS applications for medium-range weather, subseasonal to seasonal weather statistics, short-range severe weather, and hurricanes. In a new project (2024), the group is also investigating the evolving role of land-atmosphere interactions on precipitation variability and predictability from the recent past to the near future, separating the impacts of land use change and soil moisture, to determine how “hot spots” will change in response to global warming.
AOES faculty member Stan is co-leading the effort of developing the UFS applications for medium-range-weather and subseasonal-to-seasonal time scales. Her research group is evaluating the impact of tropical SST biases onto the forecast skill of surface weather over the U.S. She is also leading an international collaborative effort to develop a diagnostics package for evaluation of MJO Teleconnections (Stan et al. 2022 and https://doi.org/10.1175/BAMS-D-21-0130.1).
AOES faculty member Cash is leading multiple projects in support of development of the new, UFS-based seasonal forecast system. This workfocuses on the impact on prediction skill of ensemble size, model configuration, and source of initial conditions, among other considerations. Our group is also testing the impact of intervening in the model to reduce errors in the three-dimensional atmospheric heating field, particularly with respect to the association with tropical sources of predictability such as ENSO. We are also conducting rigorous statistical comparisons of the relative skill of the UFS versus existing seasonal prediction systems, such as the North American Multi-Model Ensemble.
Midlatitude Variability on Intraseasonal to Seasonal Timescales
Variability of the climate system as a whole is characterized by a continuous distribution across all frequencies/timescales (Hasselmann 1976) with salient features at regional scales. The midlatitudes are characterized by a pronounced seasonal cycle of weather types driven by the annual insolation forcing, internal dynamics of the atmosphere, and tropical teleconnections.
AOES faculty Stan and Krishnamurthy (2019) show that, similar to the tropics, the midlatitudes are characterized by variability on intraseasonal time scales (10 to 100 days). This variability manifests as a nonlinear oscillation that propagates in the zonal direction around the globe and meridionally in certain regions. We are focused on:
- developing and applying data adaptive methods to isolate these oscillations in observations and model simulations;
- designing and conducting numerical experiments for explaining the mechanisms that drive the variability of midlatitudes on these timescales;
applying their source of predictability to improve the forecast skill of weather prediction on subseasonal to seasonal time scales.
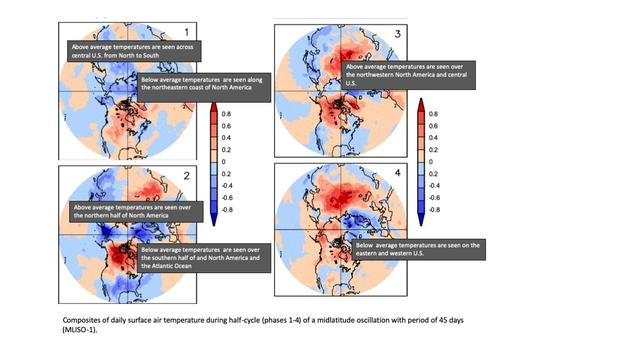
Land Atmosphere Interactions as a Source of Predictability
The slowly evolving states of the land surface are a source of predictability on a range of time scales. Anomalies in soil moisture, snow cover, vegetation phenology (greenness, leaf density, plant height) and soil temperature can affect the atmosphere above. In a forecast, the effects are felt as soon as the sun rises, as land surface states affect how much solar radiation is absorbed, and how it is partitioned between warming the ground, evaporating water (including transpiration by plants conducting photosynthesis) and directly warming the air near the surface. Numerical studies by Prof. Paul Dirmeyer and colleagues have shown the peak impact on forecast skill is at a lead time of 7-14 days, meaning the quality of land surface initialization and coupled land-atmosphere models directly impacts both weather and subseasonal forecasts. However, the ability of the land to affect the atmosphere varies in space and time, and depends both on the sensitivity of surface fluxes to land states and the responsiveness of the atmosphere to variations in surface fluxes.
The animation depicts how this varies around the world across the seasonal cycle: in blue areas the atmosphere is responsive but fluxes are insensitive to soil moisture variations; green areas show the opposite combination; only in red areas is there normally a coupled feedback loop whereby atmospheric temperature, humidity, clouds and precipitation may respond to soil moisture variations. However, in extreme situations like droughts, regions that are normally uncoupled like Northern Europe or Eastern China may experience land-atmosphere feedbacks, which may exacerbate drought conditions.
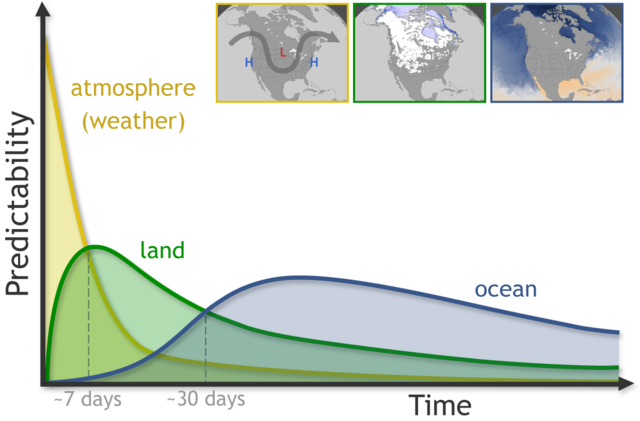
Extreme and Hazardous Weather
Predicting Extreme Heat, Rainfall
Recent research by AOES faculty Dirmeyer(https://science.gmu.edu/directory/paul-dirmeyer) has shown the effect of drought on extreme heat via the mechanism of land-atmosphere feedbacks in Europe (Dirmeyer et al. 2021 (https://doi.org/10.1029/2020AV000283)), North America (Benson and Dirmeyer 2021 (https://doi.org/10.1175/JCLI-D-20-0440.1)) and the fidelity of US forecast models to capture these relationships (Benson and Dirmeyer 2023 (https://doi.org/10.1175/JCLI-D-22-0447.1)). The clear link between water in the soil and temperature is but one example of the intertwined nature of the global water and energy cycles. AOES faculty Kinter (https://science.gmu.edu/directory/james-kinter) and Dirmeyer(https://science.gmu.edu/directory/paul-dirmeyer)https://science.gmu.edu/directory/paul-dirmeyer have a new project (https://cpo.noaa.gov/noaa-climate-program-offices-cvp-program-awards-1-8-million-to-improve-u-s-precipitation-biases/) to improve forecast model precipitation biases by investigating the evolving role of land-atmosphere interactions on precipitation variability and predictability in the recent past, present, and near future.
Flux tower monitoring indicators of extreme heat and drought over Maize in Redmere, UK (photo courtesy Ross Morrison, UKCEH).
Predicting California Drought
AOES Faculty Straus and Swenson recently completed a modeling study (Swenson et al., 2019) using both NOAA’s seasonal prediction model and the CESM model of NCAR to assess whether the very unusual California drought associated with the 2015 – 2016 El-Nino was predictable on the basis of knowledge of the ocean temperatures beforehand. Using simulations with added tropical heating (to ensure the correct forcing), we concluded that the drought was the result of intra-seasonal variability which is very difficult to predict at long range.
Predicting Extreme Precipitation Using Circulation Regimes
A new approach linking large-scale circulation regimes to the probability of extreme weather events is being investigated with specific application to the forecast of extremes by the National Weather Service at an extended range (3 to 4 weeks). We seek to determine if the forecast models in use capture the observed preferred circulation regimes in the Pacific – North American region, and whether the association of these regimes with particular patterns of extreme weather (Amini and Straus, 2019) can be exploited in the prediction of extreme weather. In related work, we are also examining the predictability of transitions between circulation regimes in the Euro-Atlantic region based on tropical forcing, in particular over the Atlantic Ocean.
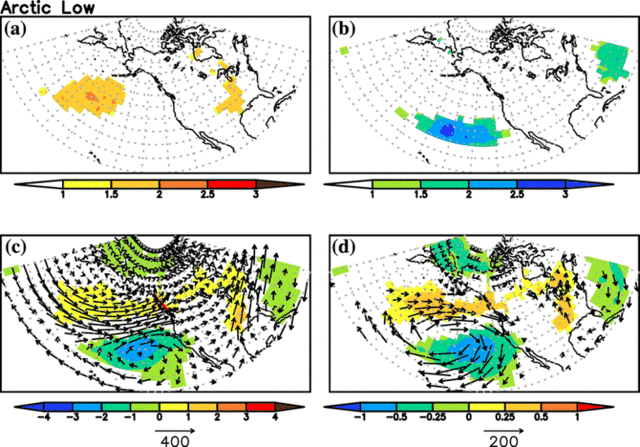
Monsoon Predictability
We are also studying the mechanisms by which tropical heating in the Indian and Pacific ocean basins influence the summer Monsoon over India. Early results show that substantially reducing the mean bias in the tropical heating in the CFSv2 model improves the equatorial wind patterns, but does not improve the forecast of summer mean rainfall. Further analysis and simulations are aimed at understanding the role of the Indian Ocean heating, and at designing techniques to improve the Monsoon.
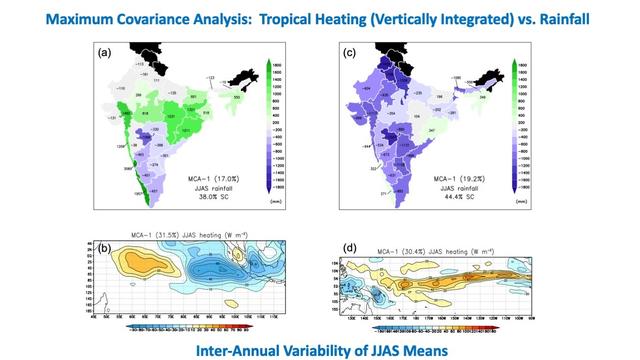
ENSO Predictability and Prediction
ENSO Re-forecasts
In the past several years, B. Huang, J. Zhu, C.-S. Shin, J. Kinter, J. Shukla, and collaborators have been conducting a series of long-term seasonal reforecasts using the US operational seasonal forecast model. These reforecasts help us better evaluate the seasonal prediction skill and predictability of El Niño and Southern Oscillation (ENSO), the strongest interannual variability in Earth climate. For instance, our results show that, given the uncertainty of current ocean initial state, an ensemble prediction initialized with multiple ocean analyses from different sources can improve the skill and reliability of the ENSO prediction (Zhu et al. 2012). We have also extended the predictions to the pre-satellite era and generated a set of seasonal reforecasts spanning the past 60 years (1958-2017) (Huang et al. 2017). This dataset further allows us to study how well we can “predict” the historical El Niño and La Niña events before 1979. In fact, our results show that many historical ENSO events (e.g., the 1972-73 El Niño) can be predicted as well as the current ones although the oceanographic observations were more limited at the time. This poses the question on whether we have fully used the more abundant measurements in current observational networks in our ENSO prediction.
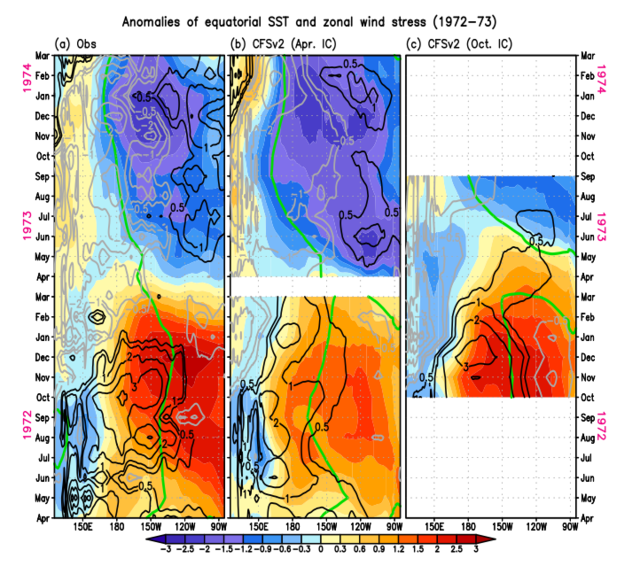
Using Machine Learning and Artificial Intelligence for MJO prediction
The Madden-Julian Oscillation is one of the engines powering the climate system on subseasonal time scales. Despite of the MJO’s pivotal role in the climate system, there remain significant gaps in our understanding of its underlying mechanisms. Consequently, climate models struggle to accurately reproduce the observed characteristics of the MJO and forecast systems face limitations in predicting the MJO with skill beyond a two-week lead time. The recent advancements in machine learning (ML) applications in predicting geoscientific phenomena spanning from weather to climate hold the promise of enhancing the skill of deterministic and probabilistic forecast of the MJO. In collaboration with the National Argonne Laboratory, Stan and her collaborators have developed a probabilistic ML model for forecasting the MJO amplitude and phase. This model harnesses the power of Gaussian Processes methodology, enabling not only to make predictions but also to quantify the inherent uncertainties associated with the forecasts.
Evaluation of subseasonal variability in the S2S forecasts is limited by the model’s inability to maintain a high forecast skill for long leads. Using ML techniques, Stan’s group is developing signal processing methods (Stan and Mantripraga, 2023 and https://doi.org/10.1175/AIES-D-22-0079.1) based on convolutional neural networks.
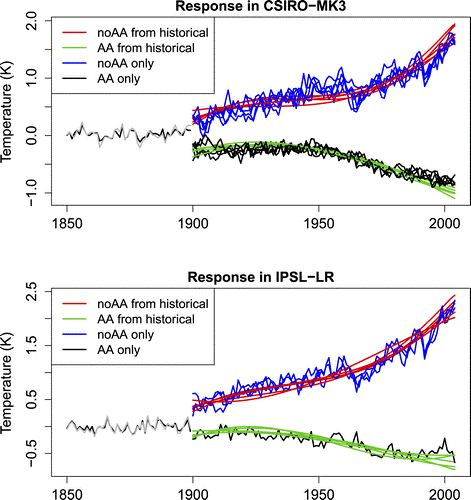
Student Opportunities
Student opportunities described below; contact the indicated faculty for details.
Undergraduate Research Opportunities
Undergraduates may work with faculty on the above research projects, or on projects developed independently by the student, supported by OSCAR, or as independent research credits.
Graduate Research Opportunities
Graduates may work with faculty on the research projects with support from a GRA (when available), a College of Science GTA, or a successful Graduate Fellowship.